
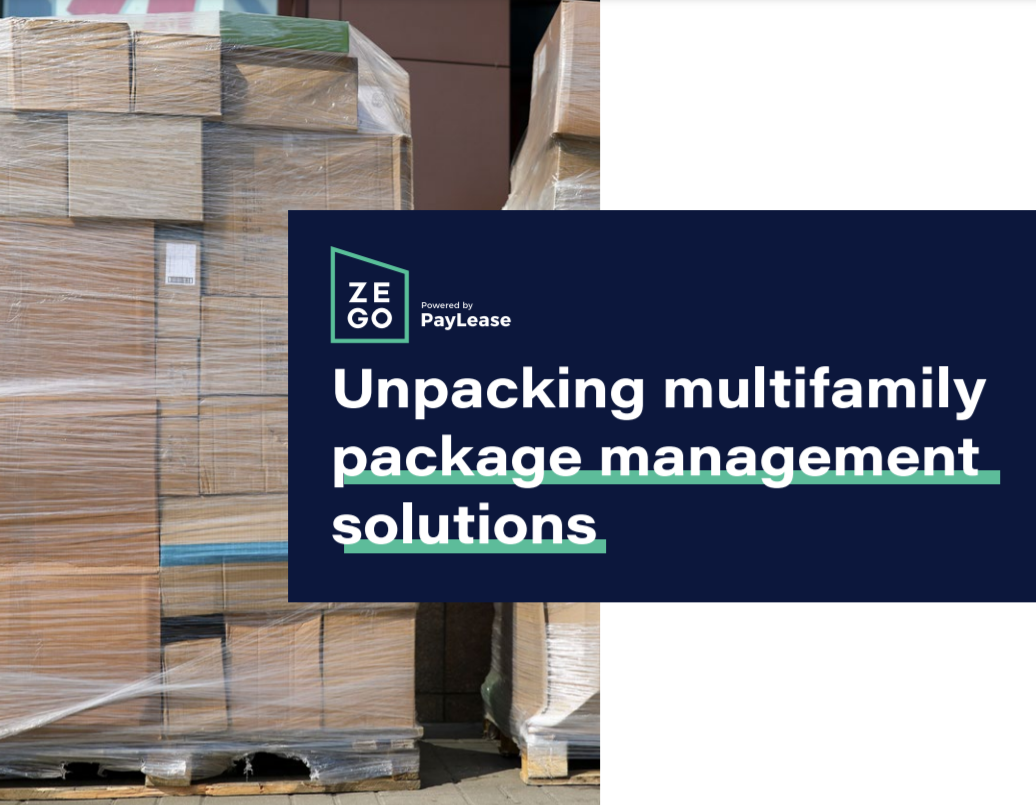
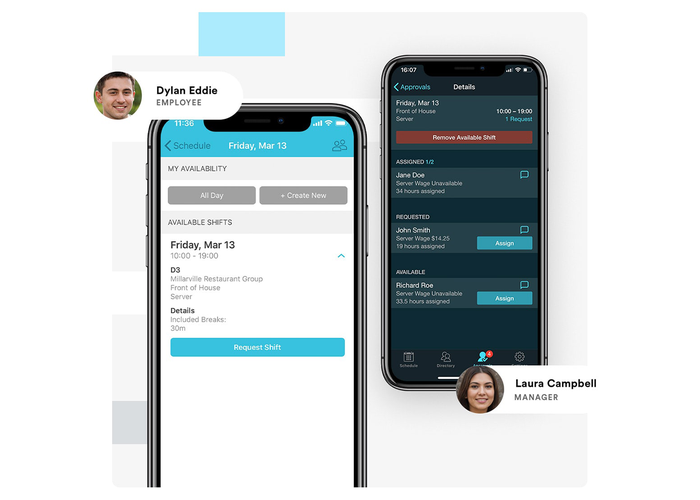
Hence, it’s sometimes hard for organizations to balance between the investment they have made for data lakes and the need to support business decision analytics to provide a single source of truth.

Since the data lakes’ schema-on-read architecture does not have a predefined schema structure in place, it’s hard to ensure that the data models are optimized for the downstream BI consumption. As a result, they lose the rich management features from data warehouses, such as ensuring ACID transactions in data updates, data versioning, and indexing. The continuous engineering effort to ETL data is necessary but may cause lots of challenges to enforce data quality and governance, e.g., querying historical data and streaming data simultaneously.ĭata lakes manage data as “just a bunch of files” in semi-structured formats, making it inadequate to support some of the key data management features that simplify ETL/ELT in data warehouses. Hence, making it difficult to support high performance decision making and BI systems. Keeping the data lake consistent is difficult and costly. Poor data quality and governance enforcement.It can get very expensive to store all of the data in a data warehouse. Although data warehouses can handle unstructured data, it does not provide it in a cost-effective way, especially with data growing so fast nowadays. In addition, more and more unstructured data such as images, video, audio, and text documents are now stored by many organizations and they need easy to use systems to manage this data. Reading this huge data via ODBC/JDBC is inefficient, and there is no way to directly access the internal data warehouse proprietary formats. Unlike BI queries, which usually extract a subset of data for a specific use case, predictive analytics need to process large datasets using complex non-SQL code. Nowadays, business users also want to ask predictive questions using their data, by using various techniques such as data mining, modeling and machine learning to make predictions about the future. Besides, business applications such as customer support and machine learning recommendation systems are simply ineffective with stale data, not to mention the fact that it also prevents an analyst from doing their daily analysis effectively while querying the data warehouses. This makes it critical when more and more business applications require up-to-date data.
#TIMEPLUS SELF SERVICE UPDATE#
Data warehouse architecture also usually increases data update time by having an additional staging layer for loading data using periodic ETL/ELT jobs, further delaying the whole process. The data in a data warehouse is usually stale compared to that of a data lake, with updates frequently taking days to load. Enterprises are forced to deal with the increased storage due to peak user loads and ETL usage, and ultimately causing them to pay the higher storage costs.
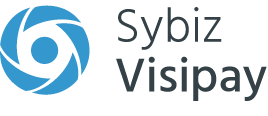
We struggle with data silos that prevent a single source of truth, the expense of maintaining complicated data pipelines, and reduced decision-making speed. Like other enterprises, we face the challenge of choosing between a data warehouse and a data lake (or more likely maintaining both!). Photo by Tatiana Syrikova from Pexels The problem
